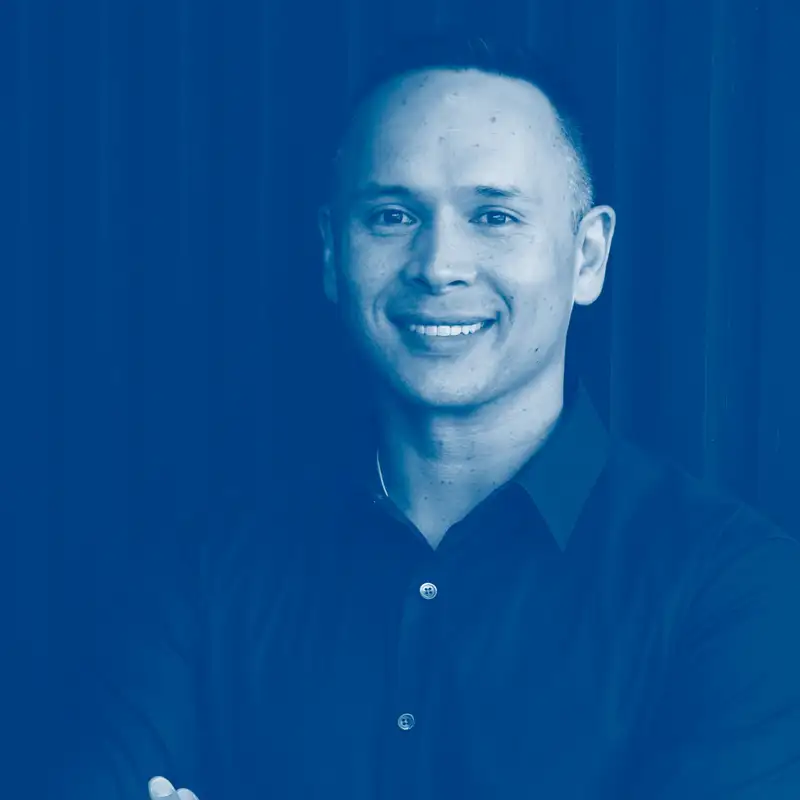
AI, VC, & Data Insights into Corporate Innovation with Thomas Thurston of Ducera Partners
On this week's episode of Inside Outside Innovation, we sit down with Thomas Thurston, Chief Technologist at Ducera Partners. Thomas and I talk about AI, venture capital, and some interesting data insights into what makes corporate innovation work or not work. Let's get started.
Inside Outside Innovation is the podcast to help new innovators navigate what's next. Each week we'll give you a front row seat into what it takes to learn, grow, and thrive in today's world of accelerating change and uncertainty. Join us as we explore, engage, and experiment with the best and the brightest innovators. entrepreneurs and pioneering businesses.
Interview Transcript with Thomas Thurston, Chief Technologist at Ducera Partners
Brian Ardinger: Welcome to another episode of Inside Outside Innovation. I'm your host, Brian Ardinger, and as always, we have another amazing guest. Today we have Thomas Thurston. He's the chief technologist at Ducera Partners. He was introduced to us from a mutual friend at Amazon, Kate Niedermeyer, who said you have a driving interest in helping corporate innovators and investors be more successful by unlocking insights from data. So welcome to the show Thomas.
Thomas Thurston: Hey, thanks. Great to be here.
Brian Ardinger: Hey, I'm excited to have you. As I've alluded to in the intro, you're a data scientist, a venture capitalist, focused on this particular space for a long time and a pretty varied background. So, tell us a little bit about yourself and what you do.
Thomas Thurston: I like to think of myself as a data scientist who's been in the venture capital industry for almost 20 years now. The idea has always been how can you use. Data, AI, any, any quantitative tools to get insights into what's happening in private markets. So, what's happening with companies that aren't disposing a lot of data that are early stage or otherwise librarial shape environment.
Today at Ducera Partners, it's an investment bank, where I'm Chief Technologist, as you mentioned. The way I would explain to Ducera which may be a little different in that it's kind of a startup investment bank. And the idea is we want to be disruptive in investment banking and really use technology as a backbone to do that.
So, through AI, through analytics we build in-house, can we do that? Can we really be disruptive in industry that hasn't seen that much change in its business model for a hundred years? And since the bank was launched about six, seven years ago, we've done over $750 billion in transactions.
We're averaging around a $100 billion a year in deals, and we've done that all with you know, somewhere around 50 people or so, although it's growing quickly. I really do think it's been the technology that's been able to enable us to really change the way we do things. So, I'm proud of that.
My story really started a long time ago when I was at Intel in an incubation group just like everyone else. They had a new business incubator, about a dozen or so projects. We were one of those projects and we were starry-eyed, hoping to build a billion-dollar business for Intel. We got our blue badges ready to go every morning. And it's kind of what you might expect the first year or, so it was amazing. We were doing great, and then one year we were super strategic.
We got this funding a few years later, we were no longer strategic, and it got shut down instead. Those decisions had nothing to do with us. So, one day someone up in top of the ivory tower thought it was optics for strategic, the next time it wasn't. And I'm pretty sure nobody was thinking about our project when that decision was made to shut it down and everything related to what we were doing.
So, I think it just was demoralizing. You give your blood, sweat, and tears to a project. At end of the day, it didn't matter, right? Something completely random blew up your project. And I just remember looking at all these cubicles at Intel and just seeing all these projects just like ours, everyone's smart, everyone's doing their best, everyone's working hard to be innovative and just wondering, does this ever work?
I mean, what? Because you know, at the beginning I thought we couldn't possibly fail. We've got this big, you know, like the best of both worlds. Big company, excitement with a startup. Couple years later, you're so grizzled and battle scarred. You're like, can this, this even possible? Because any of these projects ever work.
And I wanted to know what percentage of the time projects like this succeed or fail at Intel. And of course, I realized that nobody actually knew. Because like every big company, things get started when shut down all over the place. And it's nobody's job to run around and track it and, and kind of make a database out of it.
And if you think about the contrast at Intel, you can measure latency in picoseconds, right? They measure absolutely everything. But when it comes to all the money, I was finding in venture capital and M&A, and new product launches, kind of all this growth, money going to work, there just wasn't much in the way of quantitative metric.
It was like every other company; people do their best deal by deal. You win some, you lose some and hey, and no one could say what percentage of the time it worked. So, I just decided to start studying it myself. Spent over a year collecting data on all the product launches, all the deals I could find, to see were any of the variable’s decision makers had in the beginning correlated at all with how those deals performed 5, 7, or even 10 years later.
Brian Ardinger: Well, that's the first question I want to ask, because I think you're correct that most people don't track it or, or don't track it well. Why is it so hard to track new innovation and especially, you know, at the earliest stages? Is it because they're used to tracking different types of metrics or talk to me about that.
Thomas Thurston: It's something they're all capable of doing. But also, yet I have yet to find a big company that's actually tracking this. Usually as you know, different groups are launching new innovations or products around the company. It's not centralized, so these projects kind of get funded. They come and go.
Usually when they go, they go very quietly or they, the team gets reshuffled or something. So, there's no big announcement, and again, it's just no one's job to try to add them all up and track them. Everyone's doing it off in silos, and as you probably have experienced, there's a venture capital group. They're off doing God knows what in their thing and they're kind of behind some kind of closed door.
The M&A group is behind another closed door doing something else. They have different stakeholders, constituency. It's not centralized. But if somebody can kind of put their arms and call it data and actually start to mine it. That's the downside.
Brian Ardinger: And no one wants to talk about failure, especially with an existing company that's making money, et cetera. You know, the last thing you want to do is say, hey, my great idea was a bomb and now what do I do? Versus like in a startup world where it seems to be, if you fail, that's part of the natural process because there are things that fail when you start new things.
Thomas Thurston: Yeah. In big companies, especially. We've worked with lots of them on these issues. One thing is again, that the data's just not there. No one's collecting it. But then you do find that a lot of times it's being hidden or parts of it are being hidden by people. Again, if you're a vice president and six years ago you were part of some project that failed, you might be worried about that. Embarrassing.
In other words, if you start digging around companies, archives to try to find the history. Sometimes you realize there's people who want to bury that history. Yeah. And I don't know that they even need to, to your point, I think failure is a lesson learned and okay. Politics and big companies sometimes make it even harder in fact, they're blocking people from doing kind of work, sometimes. Not, not all of them.
Brian Ardinger: So, you started digging into this, looking at, you know, different companies and or different projects that failed or, and or, how do people kind of track this? Where's it landed? Where are you at now when it comes to this particular topic?
Thomas Thurston: What we did is we actually found a lot, and this would've been around 2007 or so. And because no one had really looked at this data, and it took a long time to even go through old Word docs and PowerPoint docs. So, I was playing anthropologists. I was having to go around the company, buy people coffee, say, hey, I heard Intel did a camera in the nineties. Someone said, you were in that project, you want to meet and talk about it.
And he goes, really just manually trying to do this. Put it in shared drive and manually create fields in a database from these old docs. And a lot of it is incomplete. So, it was really messy, but it was a passion project at the beginning. But once I had data, there were just a lot of low hanging fruit. You could just realize a lot of things that were weren't correlated pretty quickly. And since no one looks at this, every discovery was null.
But at first, I got excited, and we even found that a lot of things we looked at, our investment committees weren't correlated. A lot of the normal things we seen companies look at. There were some other things that were much more correlated with the kind of outcome we wanted. And so, there was this eureka moment, oh my gosh. Because I thought even if we get 1% better at this, at a company of that size, that's more than my salary.
So, I ended up leading a research project. I was at Harvard with Clay Christiansen for a year, Intel and, and Harvard paid for that work to be done, to really expand it with more data, bring more thought leaders in with the project. It just was so exciting for me that after that, when I left Intel after Harvard, WR Hambrecht was a venture firm where they let me keep building out the team and the technology and now at Ducera. So today it's a large team. We've got, well, $25 million just in our compute infrastructure. It snowballed quite a bit.
I think some of the, the early discoveries, so back in the 2000, 2008, first of all, we found that no matter how we defined the team, we couldn't get the team to correlate with outcomes and we defined outcomes every way we could.
So, there's all these debates as you'd imagine. Okay. What is success? So, we just tested everything we could think of and test the teams too, every way we could think to test them. And we even looked at a lot of metadata and secondary data. A lot of researchers have studied this, and nobody's apparently really has found the team has a huge impact on performance, which is shocking because one of the main things, of course, every VC looks at is the team.
We don't know what to do with that at first. The team is really hard to figure out how to correlate the team performance. Some other things that won't surprise you., we found that things like your IP, your patent portfolio, it, it doesn't really correlate. I mean, it's good to have it. You, you should definitely file and protect yourself, but it doesn't mean you're more likely to be a big success.
Of course, we didn't find financial forecast that could be correlated at all. You know, someone said that there's been more fiction written than Excel than in Word, right? Yeah. We, we found that to be true. And really what the biggest driver of the variance had to do with the competitive environment. So, we actually found that where your business enters the market, the kind of competitors you do or don't go head-to-head with had the huge impact on whether they're likely, likely to survive or fail.
Brian Ardinger: And do you think that applies to, obviously corporate innovation, but also in the startup space? Do you think those metrics as far as founding team and or market they enter. Do you think they are fairly correlated, whether you're an existing company trying to spin up something new or a brand-new company trying to spin up something new?
Thomas Thurston: Yeah, we actually found that they apply very little variance to startups as well as corporates. So, it's just as true in either case. And a lot of this goes back to Clay Christiansen and of course disruptive of innovation. Stuff he's very famous for. But I, I think we just found similar patterns in our data. And of course he was already famous for. finding disruptive innovation. And, and it was obvious that we were looking at the same thing from different angles, which was why we ended up working together. If you launch a new business with a better performing product, your odds are somewhere in that neighborhood of 13%, so very, very low right off the get go.
So, it's never go to a new market with a better product. is one of the things we learned, was statistical kind of matter, but that's pretty theoretical all by itself, right? I mean, by far the dominant strategy we see from companies and startups is to go into a market with a better product. It's what we would all assume one should do, right?
And you find out what customers want; you'll deliver a better product. But what that does is it creates a dynamic where you start, if you, if you can actually do it, you start taking customers away from the competitor, the incumbents, and it forces a response. I think the odds show it's just easier for an incumbent to defend an existing council, than for a new entrant to show up and take away the capital.
But yeah, if your odds are only 13% with a better product, that's really bad thing, even just for innovation. Normally about a third, of businesses survive 10 years, and so this is much lower than that.
Brian Ardinger: So, the goal would be potentially to either define another market and or go after a different area that you're not quote unquote good at this point, but there's more upside, more opportunity, in a new space.
Thomas Thurston: Yeah, you've got exactly right. Either find sort of a blue ocean, you know, whether there's no incumbent can be very specific, but directional. That's it. Or again, come in at the bottom of the market for cheaper and worse. And believe it or not, your odds can go up five or six x just by picking one of those different strategies.
So as an actuarial matter, you can look at businesses what their strategy is, even though they haven't executed yet. And based on that, you can sort of rate them like an actuary would. Okay, well these are the odds that you're going to hit my goals at the BC or not within the timeframe that I care about. But to do this, we have to use simulations.
So, we'll take the startup, we'll harvest all this data about their competitors. We will run all these iterative simulations to see what percentage of the time the startup, gets the kind of outcomes we want. If it's too low, we won't take the bet. Good enough. We'll keep talking. Maybe do a....
Brian Ardinger: And when you define competitors in that particular space, is it competitors who are maybe not doing the same thing, but they are solving a problem for a customer in, in a different way? Or how do you define competitors? If it's a blue ocean, for example?
Thomas Thurston: Yeah. Well, if it's truly a blue ocean, there aren't any. That's the holy grail, right? Where you, you create a brand-new market, and the key is you're not taking customers from anybody.
And that's really the best litmus test. If you make a sale, the takeaway from anybody else, is…. And if the answer is no, you're much more likely to be, we're the blue ocean and we would look at things and we call a market creating strategy. What we found is no matter how much better you are, so if you go in with a better strategy, we found it was insensitive to a lot of things. It doesn't matter how much better you are.
So, we thought, well, what if you're a hundred x better? What if you're a thousand x better? We found, nope, actually your odds are still somewhere around 13%. The other thing we looked at was price. So, what if you're better and cheaper? Nope. Turns out that they were just the same, about 13 to 14%.
We looked at spend, what if you're willing to lose a lot of money or you raise a lot of money? We thought it was insensitive to spend. So, we're really stubborn statistic kind of baffling so. And I think we really worked to find the causality and figure out what to do, but it's just kind of amazing. I mean, it almost doesn't matter. You go head-to-head with an incumbent, you're small, they're big, you're unstuck. That that's basically it. Yeah. So, find another spot at as a beachhead, that's all, but....
Brian Ardinger: Doesn't necessarily mean you can't start a company or have some success, but you won't be the outlier success or be able to tackle the incumbent the way you were hoping as introducing your product, et cetera.
Thomas Thurston: Yeah, I mean you can, 13 of them percent, 13% did survive. So, there was a chance. But if you're that sort of that blue ocean or the low-end disruption, your odds of survival are more between you know 60-65%. So, it's just as an investor, as a repeat player, you know, we just have to go with the odds. We can't bet against them and expect to be the benchmark.
Brian Ardinger: So, looking at this data, you were kind of early in this space 10, 15 years ago, taking a look at some of this kind of stuff. Obviously now, the data landscape has changed with AI and other things. How are you seeing this play out both either from an AI perspective or, or just data analysis of how companies are approaching this and or what are you doing or how are you leveraging the new data or opportunities out there?
Thomas Thurston: I think it's two interesting questions. One is, what are we seeing with companies where they're trying to use AI to make these kinds of strategic decisions for investment? Versus our firm. I mean, obviously at our firm we're fully bought in and we're just very hungry, right? We're just taking every advantage to improve and it's taking us to new places and new questions.
It's an interesting science trajectory that, you know, to me that that's super fun. But if I think about what companies are doing, obviously it's a lot slower and I think that a lot of times with a big company, it's not even about being enlightened, sort of knowing what to do. It's getting everybody else to buy in more often than not. Putting some structures in place to, to be able to execute.
So, for example, we spent a lot of time with companies years ago, they were interested in what we were learning because they had their innovation pipeline and they too, typically 10 to 15% survive on it. A lot of companies said, okay, can you help us learn what you've learned?
So, we would do that, and we would show them what we learned statistically even talk about in great detail. But a couple years later, they still didn't have better yield. The projects were still dying. And what we found is there's another causality for companies. It's a little harder to fix. It's not impossible, but it's just, and this won't surprise you, but I, I'd love to get your feedback.
Obviously, some of these really disruptive projects coming out of companies don't fit neatly into their parent company. Now they're so different, they just don't fit. You know, if you're a bank and you come up with some really interesting thing, it may not fit the bank, right? It's just too different and you need to give a lot of autonomy.
And we just find that big companies know this. That's why they have incubators, accelerators. They've tried all these things, but over the last 30 years, all these companies have been trying different, and it's still not increasingly dealt with numerically. And we figured out why and kind of what do you do instead? But it's just then that they haven't created enough autonomy for these projects.
They haven't gone all the way out to creating fully out subsidiaries and structures like that. They're all still trying to do it inside the corporate entity, which just creates too much risk and liability. That's a bigger problem though, right? Structural autonomy and a governance and a, and a wholly owned structure. Those aren't just enlightenment like Yeah, we get the board and the C-suite, they're buy in for that kind of a change.
Brian Ardinger: Do you think that comes down to a lot of like the incentives of trying to capture and maintain that value that they're creating versus it would be better and faster if they separated it out and had the value creation outside of the company. But they're either fearful or protective of what they've incubated. What they've invested to try to maximize what they put into it to get the maximum outcome. But by doing so, they actually hinder the company.
Thomas Thurston: Yeah. You got it. And I think if they keep those fully owned. Then, okay, they limited their risk. So, let's just say you have a new project in your incubator, and it's just so different. You know, to create a wholly owned subsidiary that you, you still own, you give it decent IP rights, you move the team, the project in there, they get their own business cards, but they might even be sitting in the same office as your corporate headquarters. Right.
Now as a company, what have you given up? You can still shut it down anytime you want. You can still fire everybody. You can still kind of do what whatever you want with that. But by creating a separate entity and putting the right governance in place. Now if this project goes off, first of all, the team will be delighted that now they can get a website, they can get their own CRM, they can do whatever they want.
They don't have to answer the corporate overlord kind of stuff. You freed them up to run their business. But the reason they're allowed to be free is because by creating the right structures, you've now protected the parent company from both legal and reputational risk. And so, the reason these incubators.
Typically, haven't worked is because they haven't protected the parent company. You can't free the project until you protect the parent. That's the order of it. So, if you can create a structure where you have wholly owned subsidiary and the right governance to protect the parent. Because no big company can have a hundred projects doing God knows what, running all over the world, creating liability.
Right, right. It's untenable, but it, those are encapsulated. Well, there you, there you go. You can do that. We actually have a model that we built that actually can predict. We have about over 83% accuracy. If we look at a company project, we can, whether the parent company is likely to shut it down for internal reasons or if they'll keep supporting it.
And we just found that there's actually measurable things that can tell you with any company, like what are they likely to kill. So, these are the projects like mine that they'll fund. Usually between years two and four, they shut it down. And that whole idea is you can predict when that's going to happen. You can, first of all avoid it and maybe find different pathways where you're not going to just take a team and all our hopes and dreams, burn them out for two years and then lay them off. Right.
Brian Ardinger: Right, right.
Thomas Thurston: And that's the worst possible outcome.
Brian Ardinger: Is it a timing issue? So, I see a lot of projects die because they don't get there fast enough. Or you know, the quarterly churn of an existing company. Especially publicly comp traded companies doesn't match up well with long-term value creation. You know, most startups take five to seven years to kind of get to that inflection point. Is, is it a timing issue, do you think, for some of this stuff?
Thomas Thurston: I mean, yeah, definitely. There's timing, obviously in the marketplace, timing matters quite a bit, but in a corporate setting, this structure, if you put it in the right structure, let's say, okay, now you have fully on subsidiaries and you're still funding them every year. Every year that they need to come back for money is basically they put their head on the chopping block and say, can I keep going or not?
Right? And what you can try to do, obviously harder in some industries, but if you really drive those projects aggressively to profitability, even at the expense of growth. So, say, okay, we know you're not going to grow as fast, but it's all about becoming casual break even in two to three years at that point, once they pay for themselves you can just let them run in perpetuity. I mean, ideally, you want them as big as possible, as fast possible, but if they're not coming back for money, you've essentially now got a free option of the company where if they become successful, great. If they don't, it's not costing you anything. And if you decide you're out someday, you could still potentially sell them or take external capital. You have all these choices now.
Brian Ardinger: That probably also then depends on the types of companies that you are building. And you know, for example, if you're building an internal company and it's competing against an externally highly VC backed industry, for example, that is just throwing capital at growth and they're never going to be profitable or going to be profitable in, you know, 5 to 7, 10 years, and you're focused on getting to profitability at a a much shorter time span, I would imagine competing in those particular markets is much more challenging than others. Is that what you think?
Thomas Thurston: You're right. Yeah, no, it's easier said than done. And even another example of where it's tough, especially capital-intensive businesses, for example, I mean, so they're, they're the examples where it's, it's really hard to do. And every company, you know, it is just going to be very case by case in terms of how far they're willing to go.
But I think the moral of the story is, until they can pay for themselves, until they don't need you, they're at risk. So, can you get them to pay for themselves? So, they bought a new lease on however long that s-Curve is going to need to be.
Brian Ardinger: Okay. Any recommendations for how folks can begin to experiment or start using data and AI and that and to explore if they're not already doing so?
Thomas Thurston: There's the, the small version of that. And then the big, I mean, again, for us, we've been chipping away at this for a very long time. You know, we've made major commitments to AI and all of it. We haven't personally found a ton of vendors that have been helpful. I mean, we're, we don't have any pride in terms of our firm.
Like we're just about the results. So, if we can find someone doing it better, it's the best day of my week. Because I mean, I'd much rather outsource something than build it frankly if it does the job. So, there are a ton of great vendors, the whole, the kind of insights we need in private markets. But I think one is just looking, always seeing who's out there. We do that too. Is there anything else we can use?
Hard is trying to build it in scratch, but. I would encourage, it seems a little silly, but I think every big company, there's no reason why they can't get one person, part of their job or, or even an intern to go around and find out what's been done, do what I did, do some anthropology, find all these projects. You can put it in Excel. It doesn't have to be ai. Just know what have we done, what's the success rate or not, and do the winners have anything in common? It doesn't even have to be machine learning. We just look at it and say, oh. I've never seen a company do that. Even with 30 projects, not even a database, so to speak.
I've never seen a company do that and not actually find one or two nuggets that profoundly move the needle. It's, it's just sitting there, and it just takes someone willing to be an anthropologist for as long as it takes, you know, six months to a year. Part-time. They just try to find out because until you can find out what you've done, what we find its companies are very good at repeating the same cyclical patterns.
So, start an incubator. First few years are glorious, and then few years later, nothing happens, and they shut it down and all those are terrible. And then two years later, they forget. It's amnesia. They start over. There's some new exciting right in the room. Right? And you can't break out of that unless you start to create some of that historical institutional knowledge and codifying it. Right? So, it's not just. Oh, I was there. I know. You know, it's no, no, actually when we do x we get y. Period.
Brian Ardinger: That makes sense. That's great advice and, and hopefully other companies that are listening here, will, will take a, a little nugget and at least if nothing else, just start the journey of understanding where they've been and hopefully using that to influence where they're going. The last question is, what are you excited about? You're looking at a lot of different markets, a lot of different things out there. Are there any particular trends or technologies or other things that you're excited about?
Thomas Thurston: So, our newest fund is on ag, on agriculture, ag tech. So I'm diving head first in the world of agriculture. So, I'm, I'm going to be heavily biased. That's what I've been excited about lately in terms of the investment side. It's a relief as a human being, not even as investors, to see how many people, I mean, are like solving really important problems in agriculture and food. So, thank goodness people are working on that. There is a lot of excitement.
And of course, I think AI is having this moment, again with GPT and so forth, but I think it's really accelerated the use of AI in non-AI domains, right? And there's tons of companies trying to use AI in pharma to find drugs. Tons of companies trying to do it in material science to find new polymer. There is all this going on, but that's been really slow and rough for a lot of those companies until now. And I think we're all sort of realizing collectively that, oh, there are use cases for ai, even Chat GPT or whatever, those across all these various domains. There's a lot of low hanging fruit now, so where are they now? And we'll start using them.
So, I think that's just exciting as a human being right now. I've been up to my eyeballs in AI for a very long time. It was so frustrating to try to explain what I do up until about a year and a half ago, right? Because people would just think, oh, that sounds like black magic, black box. Impossible. Computers can't do that, right?
And there's nothing you can say about that if people just can't, their belief barriers so high. But now everyone's tried chat GPT and like, oh, computers can do all kinds of things that we thought were impossible. So, it's really helped move the conversation along. I'm grateful for that. Just in terms of the zeitgeist shift.
For More Information
Brian Ardinger: We are definitely living in fascinating and insightful times. So, Thomas, I appreciate you coming on Inside Outside, Innovation, and sharing your insights and that. If people want to find out more about yourself or Ducera partners, other things, what's the best way to do that?
Thomas Thurston: Hey, they can just email me or get in touch. It's piece of cake. I'm always happy to share anything we've learned. Look, part of the fun, even like today, just being able to talk about the thing that I'm really passionate about, meet other people, believe me. Otherwise, I'm just sitting in my cave all day long, so it's wonderful and I'm happy to do it.
Brian Ardinger: Thomas, thanks again for coming out of your cave. Appreciate you being on the show and we'll, we'll look forward to talking to you again soon.
Thomas Thurston: Hey, thanks for the opportunity. Appreciate it.
Brian Ardinger: That's it for another episode of Inside Outside Innovation. If you want to learn more about our team, our content, our services, check out InsideOutside.io or follow us on Twitter @theIOpodcast or @Ardinger. Until next time, go out and innovate.
FREE INNOVATION NEWSLETTER & TOOLS
Get the latest episodes of the Inside Outside Innovation podcast, in addition to thought leadership in the form of blogs, innovation resources, videos, and invitations to exclusive events. SUBSCRIBE HERE. You can also search every Inside Outside Innovation Podcast by Topic and Company.
For more innovations resources, check out IO's Innovation Article Database, Innovation Tools Database, Innovation Book Database, and Innovation Video Database. Amazon Affiliate links for books. Transcripts done through Descript Affiliate.